AUTO LABELING
What is Auto Labeling ?
Auto labelling may be a game-changer however it required significant human intellect. Like most AI powered solutions, it requires creativity and iteration along the thanks to successfully generate time and resource savings. Using these features saves annotation time but you’ll still need to perform internal control checks on the work that’s done.
Automatic data labeling processes have the potential to beat a number of the challenges presented by the laborious annotation cycle. After training from a labeled dataset, a machine learning model are often applied to a group of unlabeled data. The model should then be able predict the acceptable labels for the new dataset. Automated data labeling algorithms are often improved via human input. After the AI has labeled the data , a person’s annotator reviews and verifies the labels. Accurately labeled data can then take its place within the training dataset. Auto labelling may be the annotator observes mistakes within the labeling they will then proceed to correct it. This corrected data can then even be wont to train the labeling AI.
The Auto-label AI is capable of handling the bulk of easily identified labels. This has the advantage of greatly speeding up the initial labeling stage. However, automated data labeling still produces a big amount of errors that would prove costly when fed through to an AI model.
1) Pre-annotate some or all of your dataset. Workers come behind the automation to review, correct, and complete the annotations. Automation cannot annotate everything; there’ll be exceptions and edge cases. One must plan for people to form reviews and corrections as necessary.
2) Reduce the quantity of labor sent to people. An auto labelling model can assign a confidence level supported the utilization case, task difficulty, and other factors. It enriches the dataset with annotations and sends annotations with lower confidence scores to an individual for review or correction.
Advantages of Auto labeling.
Faster and fewer expensive
Because there’s little (or no) human intervention in automated data labeling, businesses save significant operational costs and time they might otherwise spend to rent technical experts or create an in-house team.
More precise learning and improvement
Using active learning, a semi-supervised approach, automated data labeling provides highly accurate data annotation. Active learning requires the labeler to pick an initial sample from unlabeled data then label more data based upon the results. additionally , automation are often leveraged to repeatedly enhance and improve your manual data labeling processes.
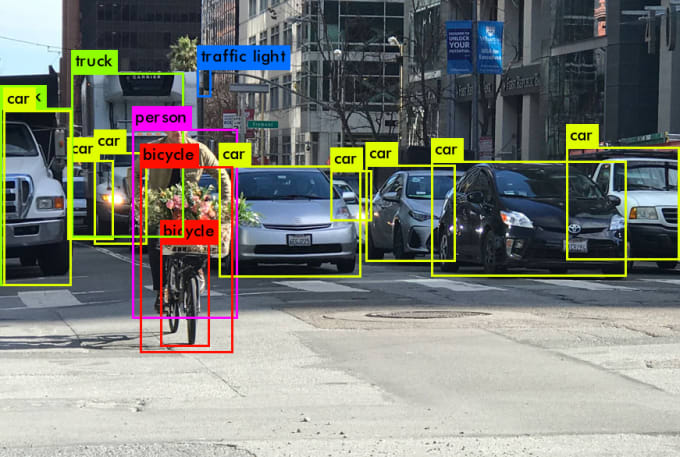
How Vancouver Automation can help you ?
Material Handling is an essential part of every automation system. Whether moving heavy awkward parts or small intricate precision components, efficient manipulation and control is essential. From the newest motor assembly to the most intricate surgical device, material handling and its flow ensures that components can be turned into assemblies that guarantees an assembly solution will not choke off critical up time or contribute to costly damage to parts or operators. Vancouver Automation through its experience along with its partners to provide technologically leading solutions that ensure the backbone of the custom equipment link together in support and harmony. There are many solutions that are tried and true and new flexible solutions that deal with the complexities in the market today. Material handling solutions range from linear solutions in layout and form too numerous to detail but include our partners: Robert Bosch power and free conveyor, belt driven solutions coupled with flexible feeders, bowls, hoppers with vision, and escapement devices that verify orientation and control throughout the assembly process.